Frank Beard is a longtime convenience retail enthusiast who currently works in marketing for Rovertown. His column, The Road Ahead, examines innovation in the c-store industry.
Hype cycles can be an emotional rollercoaster.
Remember when RFID was said to be “retail nirvana” in the early 2000s, or when 5G was bringing about a fourth industrial revolution? How about Bluetooth beacons — one report nearly a decade ago claimed beacons were the most important retail technology since mobile credit card readers.
Prior to the pandemic, there was an endless drumbeat of hype about Amazon disrupting grocery and convenience with autonomous stores. The past few years even saw folks lured into web3 scams while major retailers jumped aboard the metaverse hype train and sold digital items in ghost towns like Decentraland.
It’s of course easy to criticize hype cycles in hindsight — or go all in while expectations are inflated. The hard part is taking the time to figure out how to make these potentially disruptive technologies constructive for your business.
After all, they’re hyped for a reason. Maybe RFID did have a failure to launch fifteen years ago, but it turns out it’s very useful in apparel retailing. Even Uniqlo is making a big bet on RFID right now.
And while the autonomous retail revolution hasn’t exactly materialized, computer vision does have tremendous potential if applied to the right problems.
When Just Walk Out just didn’t happen
From 2018 to 2020, Amazon Go was a topic at every single retail conference I attended.
Bloomberg was partially to blame for the hype by publishing claims that Amazon planned to open as many as 3,000 autonomous stores by 2021, but the public launch of the original Amazon Go in January 2018 happened at a time when the house that Bezos built seemed invincible.
And yet, the disruption never came.
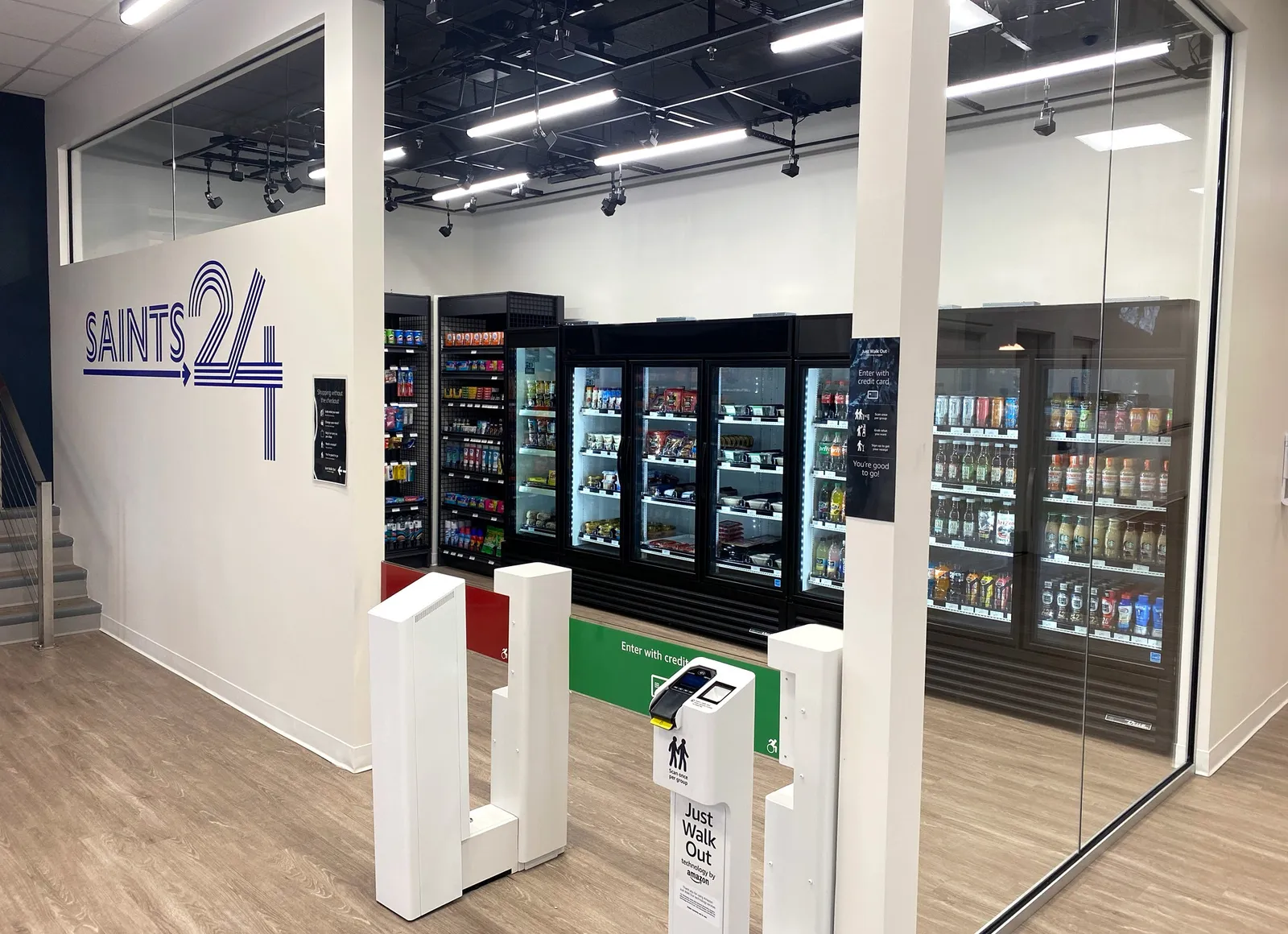
Today, Amazon’s Just Walk Out (JWO) technology is installed in roughly 20 Amazon Go stores, 40 Amazon Fresh stores and two Whole Foods stores. Despite being available to third-parties for over three years, JWO is only found in around 50 non-Amazon locations — most of which are stadium micro markets.
As The Information recently revealed, JWO also consumes hundreds of millions of dollars in annual cloud computing costs, had 2,000 employees working on it before last November’s layoffs, and as of last summer had 700 human reviews for every 1,000 sales. This means your slow receipt is likely due to someone reviewing videos to determine if the Red Bull you picked up is an 8.4oz sugar-free can or a 12oz regular can.
Retrofitting the technology into existing stores is also time-consuming. Kansas City’s Community Grocers had to shut down for seven months to install JWO — and lost $40,000 per month.
But Amazon isn’t alone here.
Its Sputnik moment was so impactful that investors poured hundreds of millions of dollars into startups building similar solutions. Of the major autonomous retail startups active in the US, Standard AI (full disclosure: my former employer) raised $239.4 million, Trigo raised $204 million, Grabango raised $93.8 million, AiFi raised $80.4 million and Zippin raised $44 million.
But AiFi, the clear leader in terms of store count, only recently surpassed the 100-store milestone despite being around since 2016. On the other end of the spectrum, some companies are opening few if any stores. Accel Robotics, which was founded in 2015 and raised $35.5 million, spent nearly a year promoting a single store on LinkedIn before its account went quiet 10 months ago.
We’ve heard for years that scale is always just around the corner, and one CEO even claimed that 2023 is “the year checkout-free shopping technology goes mainstream as more and more retailers deploy the technology across chains and regions.” However, deployments are often small pilots and press releases frequently focus on single stores.
I want to be clear that all of these companies deserve credit for what they’ve built. It’s impressive technology, and it’s a wonderful experience as a consumer. Just the other day, I had to wait behind a lottery customer at one of my local convenience stores — which is especially irritating as someone who once complained to Disney Guest Services about the length of the FastPass queues.
Would I have preferred to just walk out? You bet.
But it does seem like there are two strategic errors being made here.
An expensive solution to a simple problem
For starters, there’s been a simple solution to checkout throughput since the 1990s: self-checkout.
Are they perfect? Of course not. But any retailer who wants to optimize throughput and redeploy labor can install kiosks more quickly and at a fraction of the cost of autonomous platforms. This dynamic will be more favorable for autonomous checkout in time, but the question is when?
Encouraging adoption is also easier since customers are already familiar with the technology — and many kiosks accept a full range of payment methods, including cash. The new computer vision-assisted self-checkouts may have a novelty factor, but they’re still packaged in a format we know and trust.
This is a lesson Circle K learned over the past few years.
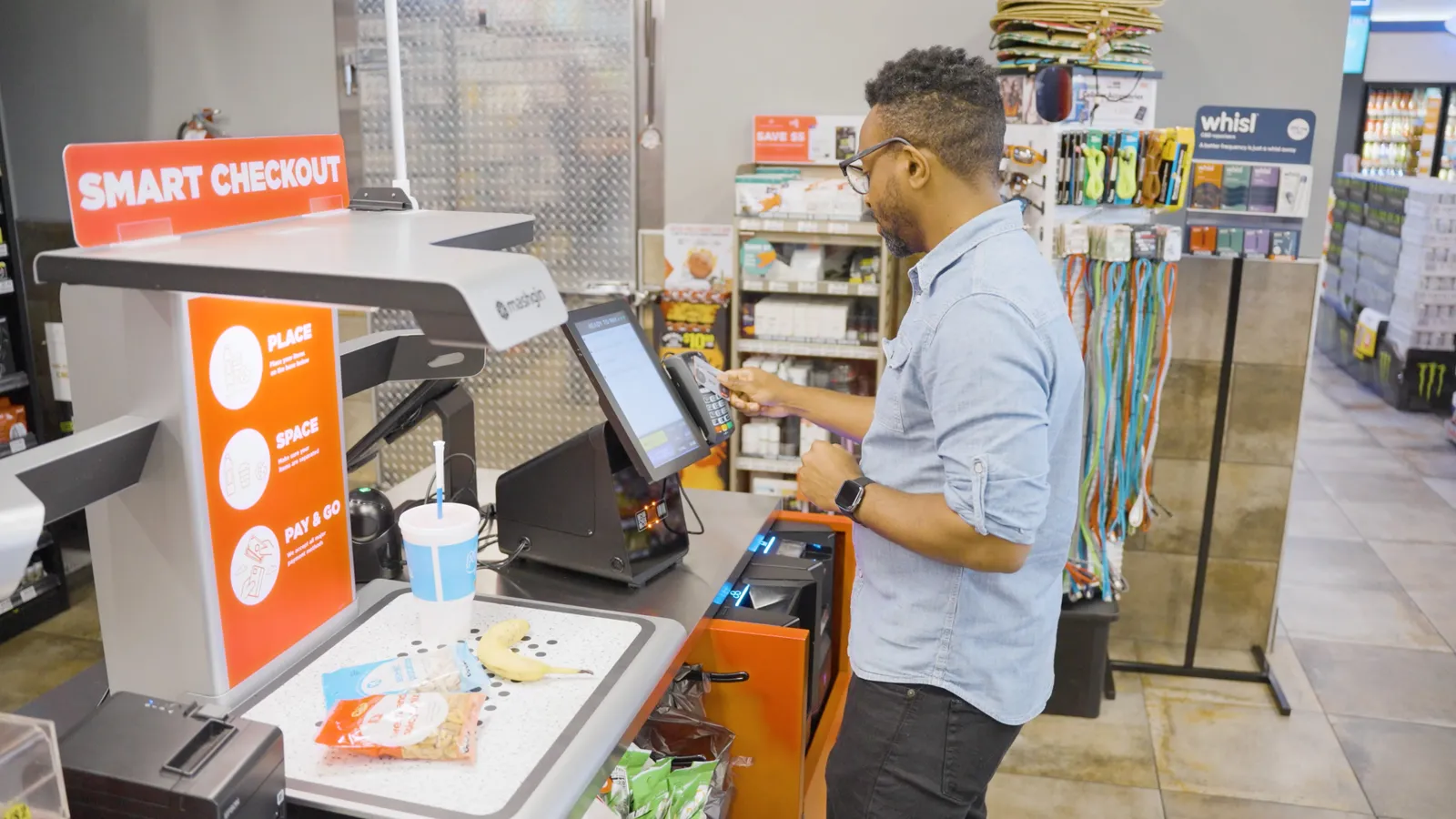
In 2021, parent-company Couche-Tard operated a small autonomous store-within-a-store concept at its retail lab at McGill University. Shoppers had the option to either download an app to gain access to the autonomous store— since access was controlled by gates, like Amazon’s JWO stores — or shop at a larger adjacent store that had cashiers.
Take a guess which option shoppers preferred.
Shortly before McGill’s first autonomous store was shut down and a second, more advanced platform was installed across the entire retail space, Couche-Tard added Mashgin’s computer-vision-based self-checkout alongside the cashiers. The customer response was so positive that Couche-Tard announced the deployment of around 10,000 Mashgin units to more than 7,000 stores.
But this leads me to the second strategic error.
Both at McGill and at autonomous store pilots in Phoenix and Tucson, Circle K — like other convenience retailers piloting this technology — led with checkout automation rather than using computer vision for operational efficiency.
Focus the technology on what really matters
What happens next with Circle K is anyone’s guess since it shuttered its global innovation team earlier this year, but I believe it missed an opportunity to apply the technology to areas of the business with more upside.
Is the difference between self checkout and autonomous checkout so meaningful that it will influence consumer decisions? Outside of isolated use cases in stadiums, I don’t believe so. Amazon has learned this lesson the hard way.
But at some level, every retailer knows their stores could operate better. That’s where there’s a real opportunity to use technology to influence high-impact decisions.
Which products should each store carry? Where should they be placed, and how much shelf space should they get? When should they be reordered, and what should the price be? How much labor should be scheduled? How should all of that and so much more differ on a per-store basis?
AI brings the potential to make these decisions better, faster, with more context, and with higher precision and lower error rates, than any human. But retailers must first know what’s on the shelves so they don’t train models with stale, inaccurate data — leading to a garbage in, garbage out scenario.
That’s where computer vision comes in. If done right, it opens the door to real-time, accurate inventory data. Or at minimum, it can be used for specific operational use cases that might pay for themselves — like reducing the ease of theft at self-checkouts — and be achieved with fewer cameras and lower cognitive load than autonomous checkout.
For anyone who wants a deeper dive on computer vision, it’s discussed in a new book on retail AI by Francois Chaubard, the CEO of Focal Systems.
But the big picture here is that convenience retailers, for the first time ever, are facing a future where there’s competition for transportation energy. Continuing to grow scale at the same 3%-6% net margins may prove to be very difficult.
That’s why I think convenience retailers would be wise to focus their computer vision pilots on operational use cases rather than seeking minor improvements in checkout throughput. There’s simply more potential to increase free cash flow. Indeed, retailers like Walmart Canada are already on this journey in a big way.
The question is which convenience retailer will be the first to make computer vision work for its business?